When I stumbled upon a website called ImageNet Roulette that categorizes images you upload, I couldn’t resist trying out a few of my own pictures and seeing what categories I would get. First, I tried out a few pictures of my cat, who was categorized as “favorite pet” and “bedlam (a woman of advanced age).” My cat is pushing 7 years, but I wouldn’t call her an old woman. When I started plugging in pictures of myself, I was mostly categorized as a “prophetess”, “grinner”, “flibbertigibbet”, “creep” or “weirdo.” I couldn’t help but crack up at these ridiculous groupings, but the seemingly silly and innocuous categories started to become more uncomfortable as I tried out pictures of me with other people. Now I was categorized as a “black woman”, “mulatto (an antiquated term for mixed-race)” and “whiteface”, possibly being categorized as different in color than the people around me.
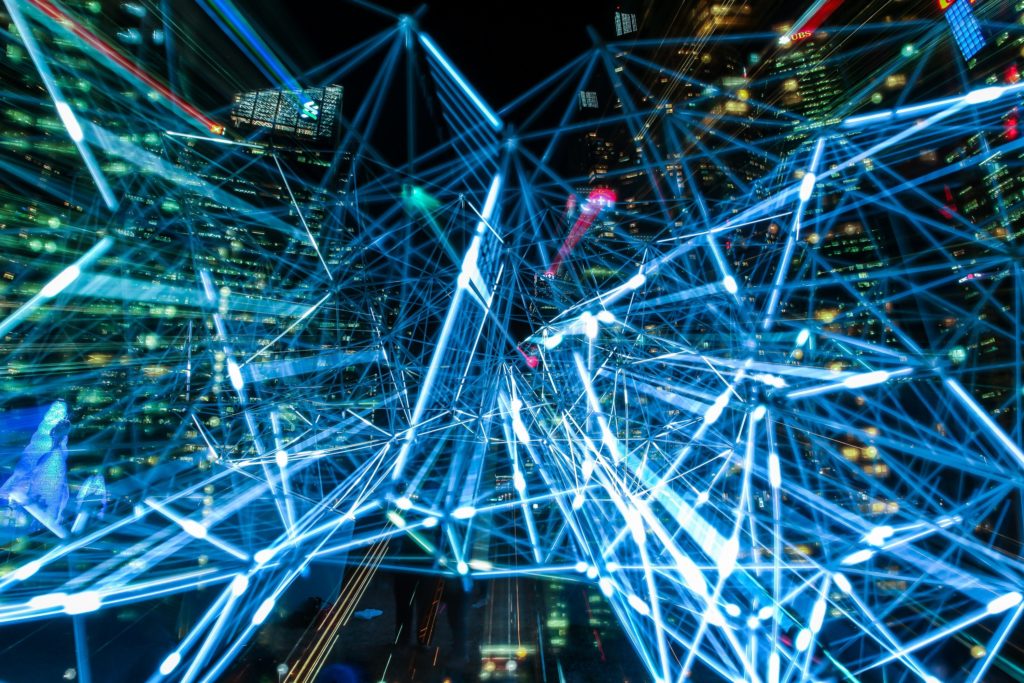
ImageNet Roulette serves as a PSA on how machine learning categorizes humans using one of the most influential training sets in AI, ImageNet. It’s meant to show how AI can go wrong when its inputs are biased, illustrated from the often racist, misogynistic and absurd categorization that can occur. This is mainly why the website has now been taken down. The ImageNet dataset is eurocentric and has limited data on the diversity of the human experience. As an Indian-American woman, I likely wouldn’t be represented in the dataset. To test this I tried an image of myself wearing Indian attire, to which I was categorized as an “astronaut,” or “spaceman.” ImageNet had no context for me, so it decided I was literally out of this world!
The idea of categorizing people is something humans do all the time; we’re exceptional at picking up meaning in images, emotions from facial expression and context in different environments. When you ask a computer to do the same thing, it tries its best but doesn’t have the same cultural, emotional or modern understanding to be able to do what our brains do effortlessly. As the creators behind ImageNet Roulette, Kate Crawford and Trevor Paglen, argue in their thesis, Excavating AI, categorization is inherently political:
“To create a category or to name things is to divide an almost infinitely complex universe into separate phenomena… Nouns occupy various places on an axis from the concrete to the abstract… everything is flattened out and pinned to a label.”
They’re not just arguing that there are inherent problems with the technology or datasets used to train AI, they are trying to convince us that the action of categorization will always be inherently political or social and therefore skewed. If this is the case, decisions on how and what an AI dataset should look like shouldn’t be restricted to academic circles or large technology companies. This is especially true considering how important these datasets are and will become in our daily lives. So in the end, do you think we can trust a computer to put a label on us? If not at the moment, what would the dataset look like if we could?
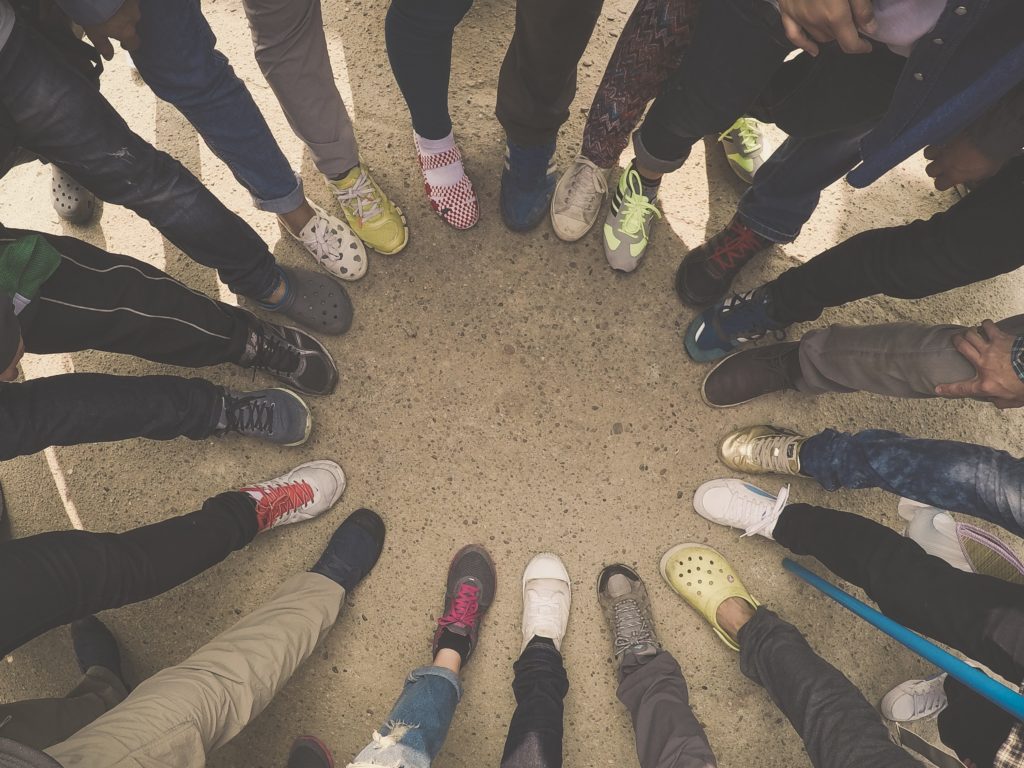
As the website has been taken down, you won’t be able to have first-hand experience with the shortcomings of ImageNet Roulette. However, I highly recommend reading the beautifully written thesis on The Politics of Images in Machine Learning Training Sets in Excavating AI.
Peer edited by Brittany Shepherd